Being human in the time of neuroscience and artificial intelligence involve carefully exploring the nexuses of complexity where valid ideas are nevertheless in tension, manifesting subtleties and challenges that must not be overlooked. Each page expresses the existing tension(s) between ideas and within each theme, which emerged in the collective discussions, and are then complemented by insights from NHNAI network researchers.
Complexity on democracy #1: What place for data and AI in public services and the management of collective life?
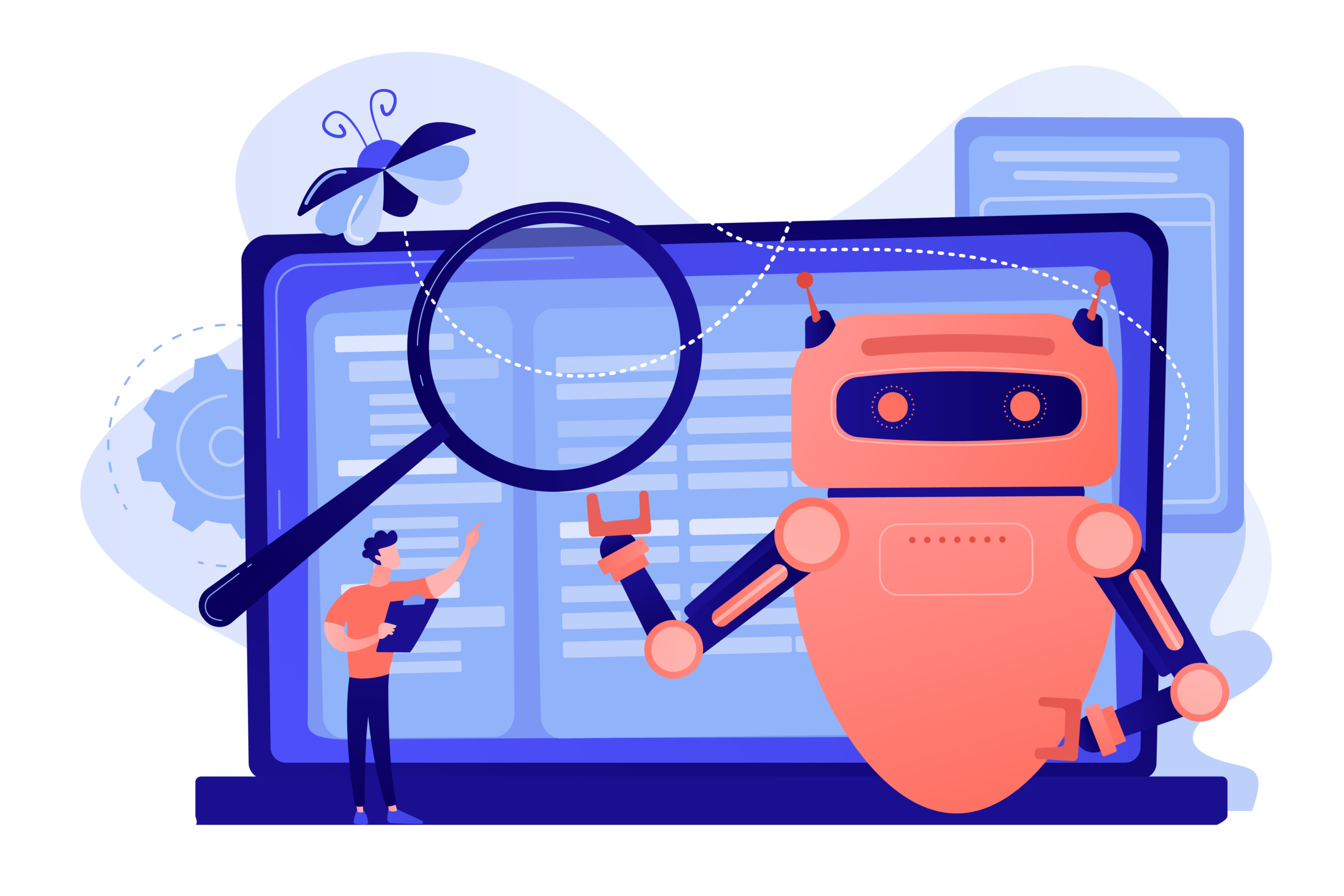
The content of the discussions shows that many participants recognize the interest of AI technologies in increasing the efficiency of public services by making them more accessible (through digitization) and more efficient (thanks to the automation of certain tasks, e.g. administrative). AI and digital technologies also seem to be seen as interesting for facilitating democratic life and political decision-making (notably with data analysis to better understand currents within public opinion).
Nevertheless, many participants also point to the importance of not pushing humans into the background, and of subjecting people entirely to algorithms. There was a lot of discussion about the importance of leaving algorithms in their place, as tools to serve and cooperate with humans (but not to replace them entirely). Collective (democratic) life necessitates to preserve (or even increase) empathy and relationships between humans. The automation and digitization of public services is not necessarily, in itself, beneficial for everyone. Some populations may find it difficult to access digital tools, and algorithms may contain biases and automate certain forms of discrimination. It is therefore important that decision-making (at political or public service level) remains under human control.
Automation and the use of data in the conduct of public affairs can therefore be a source of great progress, but must not be to the detriment of humans (or certain more vulnerable groups). Mobilized AI technologies must be reliable (deceiving hopes triggered by announcement of digitalization may undermine even more trust in governments), and display strong levels of fairness, accountability and transparency (to ensure trust-building and social acceptance).
On a more fundamental level, many participants claim a kind of right not to be reduced to their digital data.
Insights from NHNAI academic network:
Based on insights from Brian P. Green (professor in AI Ethics, Director of technology ethics at the Markkula Center for Applied Ethics (Santa Clara University, USA), Mathieu Guillermin (associate professor in ethics of new technologies (UCLy (Lyon Catholic University), UR CONFLUENCE : Sciences et Humanités (EA 1598), Lyon, France), Nathanaël Laurent (associate professor in philosophy of biology (Université de Namur, ESPHIN, Belgium) and Yves Poullet (professor in Law of new technology of information and communication (Université de Namur, ESPHIN – CRIDS, Belgium)
A. Improving efficiency of democratic processes without undermining persons’ singularity
AI may help us in many domains. We want to use AI to become more efficient at good things and at the same time use AI to make bad things less efficient. Can AI help to make it easier to help people? Can AI be used to catch corruption? What other good things can AI help with and what bad things can AI help to stop? The use of AI to reinforce democratic processes is an interesting one, also likely fraught with controversy, but perhaps capable of doing things never before possible with democracy, like giving surveys to entire populations and finding what “the people” really think about many political issues, with uncertainty bars around them, and so on. A new form of democracy might be possible. That does not mean it will be any better, but it might be worth doing a pilot study and experimenting with it.
Any effort in this sense should nonetheless never undermine the centrality of the human person (and of other living beings). A first fundamental principle that we should assert is the right for everyone to participate in the information society. This right must be progressively enlarged since more and more the use of the infrastructure and certain digital services are today becoming essential for the development of our personality. This right implies a right to education to digital literacy[1] and as well as the right to the ‘core platform services’ offered by such as communications’ social networks and search engines.
Preserving the centrality of the human person also means respecting the principle of human oversight (the control by human people of the functioning of AI systems). Moreover, people should never be integrally subject to decisions taken by automated systems. Explanations of decisions must be furnished by human people and a right of recourse must be warranted.
This respect for the centrality of the human person ties in with one of the strong axes of Pope Francis’ positioning on AI in connection with resistance against what he calls the “technocratic paradigm”: “Fundamental respect for human dignity means refusing to allow the uniqueness of the person to be identified by a set of data. Algorithms must not be allowed to determine how we understand human rights, to set aside the essential values of compassion, mercy and forgiveness, or to eliminate the possibility of an individual changing and leaving behind the past.”[2]
B. Are algorithms more neutral than humans?
With this in mind, it is important to solidify our collective acculturation to digital technology. Indeed, the notion of algorithm can easily convey the idea of an absence of bias and, the idea of enhanced rationality or objectivity by comparison to human judgment (after all, algorithms are logical-mathematical procedures that leave no room for arbitrariness or human subjectivity). Yet this connotation masks a much more contrasting reality.
The basic intuition is valid: if a discrimination is explicitly programmed, it will “show up” in the program and the programmer can be called to account. However, this transparency is not necessarily the case with AI programs obtained through so-called machine learning. Without wishing to join the ranks of commentators who present these programs as black boxes (we can watch the calculations being made, nothing is hidden or invisible on principle), it is important to understand that they can very easily include biases and lead to discrimination that is difficult to detect by looking directly at the program’s content.
Indeed, the general idea behind machine learning is to attempt to bypass limitations in our ability to explicitly write programs for complex tasks. For example, we can easily write a program to distinguish between black and white monochrome images … all it takes is a few simple calculations on the numbers encoding the color of the pixels in such images … but what calculations can we make on these same numbers to obtain a program to distinguish between multiple images of everyday objects? At this stage, we can try to go a step further by writing a program with “holes”, or rather “free parameters”, i.e. an outline of a program capable of performing many different logical-mathematical operations (multiplication by coefficients, additions, other more complex operations) and chaining them together in a multitude of ways. The details of the operations will be determined by setting the parameters to a certain value. The idea of machine learning is to say that, with a bit of luck (and above all a lot of skill and astuteness from the behalf of developers), there is a set of parameters that will produce an efficient program for the task that was resisting until now (e.g. classifying images of everyday objects). Then, we’ll try to find this famous set of parameters (or at least a satisfying set of parameters) automatically, with another program that will test a large number of parameter-setting possibilities by grouping around more or less efficiently. A very effective way of guiding this automatic parameter-setting program is to give it numerous examples of the task at hand (i.e. numerous examples of images already classified according to what they picture). If all goes well, the result is a correctly parameterized program that reproduces the examples (we say we’ve learned a model or trained an algorithm… but it’s still automatic parameterization).
C. Algorithms embed humans’ (intended and unintended) objectives and tendencies
With this basic understanding of machine learning, it’s easier to see how “successful” learning process can still lead to a highly problematic program. If we guide an automatic parameterization with biased data at the outset (reflecting sexist or racial discrimination, for example), successful learning will lead to a program that reproduces these biases or discriminations.[3] Similarly, if we “train” a program on non-representative example bases (for example, because groups or minorities are not represented in the data), it is very possible that the program will not work as well for all the persons who will use it or be subjected to it.
In general, it is very important to debunk the illusion of digital technology as mere neutral tools humans create, store aside and mobilize only when needed. Rather, digital technology, as any technology, is better conceived as networks of interrelated human actors (computer scientists, designers, programmers, engineers, users, etc.) and non-human components (servers, rare earths and lithium mines, water resources mobilized for data centers cooling, etc.). Accordingly, the behavior and outcomes of AI systems (and more broadly of digital technologies) will always result from (and reflect) what humans willingly or unwillingly made them with (programming, examples in training datasets, socio-ecological impacts, etc.).
In particular, AI will reflect, propagate and possibly reinforce power asymmetries in society. Because AI is a centralizing technology (centralizing data, computing power, and human talent), it disempowers those who are not centered. In this way, AI is antidemocratic. But democratic societies can control antidemocratic influences if they are smart enough to perceive them and determine how to keep them on the democratic “leash.” Those with control over AI need to be responsive to those who are subject to their power, whether it is businesspeople, government officials, engineers, and so on.
This means that delegating some tasks of governance to (machine learning) algorithms and AI systems can prove beneficial only if conducted with extreme caution. The point of view of Antoinette Rouvroy (Belgian philosopher and lawyer) is particularly enlightening in this respect:[4]
Machine learning and, more generally, the ability of machines to make us aware of the regularities in the world that can only be detected in large numbers, is intended to increase our individual and collective intelligence by giving us access to a ‘stereo-reality’ that is both analogue and digital, and that can improve the way we govern ourselves and coordinate our behavior in a sustainable way (provided, however, that we recognize that algorithms are, just as much as human decision-makers, always ‘biased’ in their own way, even if these ‘biases’ do not appear to be easy to detect because they seem to have been ‘absorbed’, However, we must recognize that algorithms are just as ‘biased’ in their own way as human decision-makers, even if these ‘biases’ are not easy to detect because they seem to be ‘reabsorbed’ in the hidden layers of neural networks).
In her criticism of “algorithmic governmentality”, Antoinette Rouvroy warns against the risk of a too large and undiscriminated delegation of decision-making to machines that would lead to replace our human and living ways of enunciating, verifying and justifying our convictions by “a regime of optimization and pre-emption”:[5]
The categories or forms (ideologically contestable, subjectively biased, always a little ‘inadequate’, etc.) through which we are socially, culturally, politically or ideologically predisposed to perceive and evaluate the events of the world and its inhabitants are thus replaced by the detection of signals in ‘real time’ and an anticipatory evaluation not of what people or events ‘are’, but, in the mode of ‘credit’, of the opportunities, propensities, risks, etc. that their forms of life ‘carry’. The aim of algorithmic modelling is no longer to produce ‘knowledge’, but to provide operational information that is neither true nor false, but sufficiently reliable to justify pre-emptive action strategies.
Moreover, as already evoked, algorithms must not be understood as neutrally processing facts. Facts themselves are never neutral. Humans are always endowed with the responsibility of establishing the facts, interpreting, making sense of reality. This is of course a fallible endeavor that can be perverted. But algorithms do less (and not more) than this:[6]
For algorithms, the only ‘facts’ are the data, rendered amnesiac of the conditions under which they were produced. Yet facts, or data, are never more than the reflection or effects of power relations, domination, discriminatory practices or the stigmatization with which social reality is riddled.
[1] As a striking illustration of this issue of inequalities of access to basic digital services, a recent Belgian survey pointed out that, in 2023, “40% of Belgians remain in a situation of digital vulnerability, due to poor digital skills or non-use of the internet. The acceleration in the digitization of our society is therefore not leading to a proportional increase in digital skills” (https://kbs-frb.be/fr/quatre-belges-sur-dix-toujours-risque-dexclusion-numerique).
[2] Message of his Holiness Pope Francis for the 57th World Day of Peace, 1st january 2024, https://www.vatican.va/content/francesco/en/messages/peace/documents/20231208-messaggio-57giornatamondiale-pace2024.html
[3] One example among many others (here with generative AI): https://restofworld.org/2023/ai-image-stereotypes/
[4] Interview of Antoinette Rouvroy on the topic of “algorithmic governmentality” (2 December 2019 by Catherine De Poortere) (our translation):
[5] Ibid. (our translation).
[6] Ibid. (our translation).